Research at LME
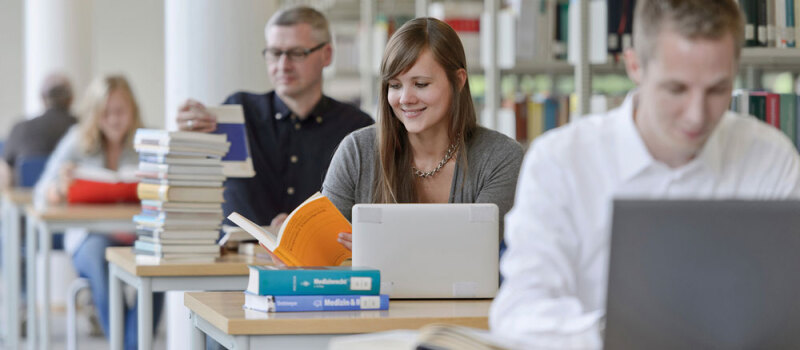
The lab combines basic and applied research from a cognitive psychology perspective with special emphasis on psychological processes relevant for digital education techniques and sustainability / protection of environment. Below you find a list of topics. For lists of publications please see the pages of the lab members
- Cognitive processes in choice, learning, and multitasking (Dr. Christoph Naefgen)
- Learning and motivation in online learning environments (Dr. E. Helin Yaban Yalçın; Monica Mary Heil)
- Learning and deciding with data graphs (Dr. Christine Blech, Dr. Daniel Reimann, Dr. Mariam Katsarava)
- Supporting communication for patients in intensive care units (Dr. Christina Ingwald)
- Information and motivation in protection of environment/sustainability (Dr. E. Helin Yaban Yalçın, Dr. Mariam Katsarava)
- The lab cooperates with the following research clusters of FernUniversität [ https://www.fernuni-hagen.de/english/research/clusters/index.shtml ]:
(1) Center of Advanced Technology Assisted Learning and Predictive Analytics (CATALPA)
(2) Energy, Environment & Sustainability - The contributes to the development and uses the Hagen archive on history of psychology: https://www.fernuni-hagen.de/psychologie/forschung/schaufenster/index.shtml
Links
Allgemeine Psychologie: LME
| 30.04.2025