News
Meet CATALPA: Adaptive learning tailored to students with APLE II
[09.11.2023]Learning platforms such as Moodle can provide very large cohorts of students with learning material and tasks. But how can these learning platforms really support students in their learning? In the CATALPA project APLE II (Adaptive Personalized Learning Environment II), Niels Seidel and his team are investigating which AI-based tools are most helpful here.
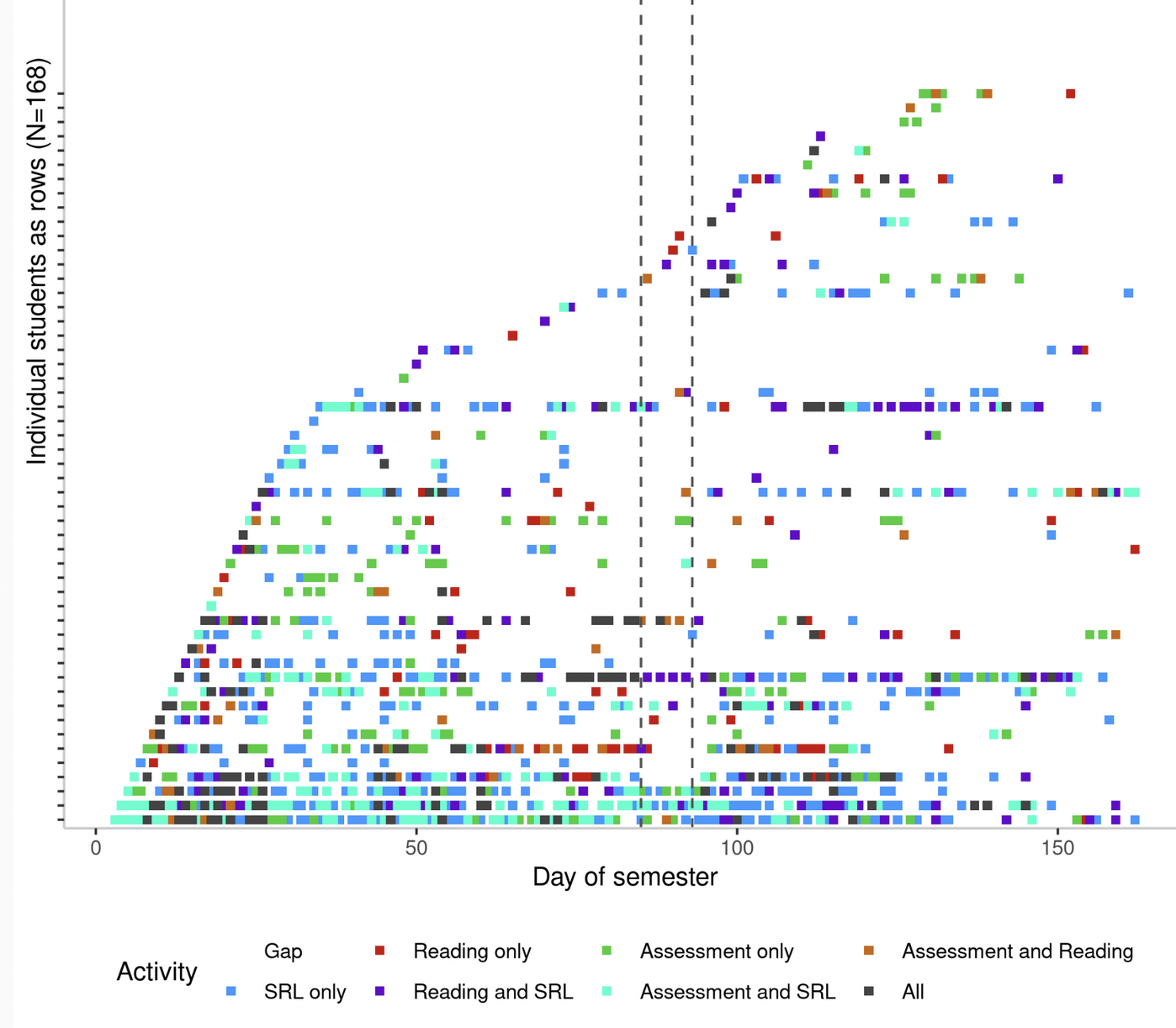
An adaptive learning environment behaves like an attentive teacher who keeps an eye on the learning progress of each individual learner and gives hints and feedback at the right time. The result is a personalized learning experience for students. It meets their needs, preferences and current learning level by focusing their attention on essential aspects of learning. In the APLE project, adaptive learning support is already being used in teaching. "Since 2018, we have implemented some courses at the FernUniversität in a research learning platform, similar to a living lab, for our research purposes," says Dr. Niels Seidel, explaining the framework for his research. Adaptive learning support is being examined in three areas:
1) In self-regulated learning courses offering a variety of learning opportunities that are meant to be used over a longer period of time up until the examination.
2) When reading extensive study texts and checking reading comprehension at the same time.
3) When solving exercises and self-assessments with the help of assessment criteria.
"In all three areas, we reflect learning behavior in the form of data graphics and make them available for the student. For example, a student can see which sections of the study texts they have already read several times and which they merely skimmed over. In the self-assessments, visualizations help to make knowledge gaps and next learning steps visible. Typical for our work in the area of learning analytics, we use so-called learner dashboards, which display learning progress and learning achievements for each learning activity at a glance. In this way, self-regulated learning is being encouraged," explains Niels Seidel, who is already in the middle of the project.
The special feature of the APLE II project is the support of learning through feedback. At course level, the learner's results for similar activities, such as reading and exercises, are summarized and compared with the goals they have set themselves. Based on this, next steps and learning strategies can be suggested. The control of the personalized feedback is in the hands of the teachers. Even if feedback texts can currently be formulated using Large Language Models (LLM), teachers can determine the situations and input parameters that are decisive for the feedback. At the level of study texts, the adaptive assessment of reading comprehension constitutes feedback per se. However, comprehension questions are selected to reflect reading progress, comprehension level and transfer knowledge within the text and in relation to related study texts.
PhD student from APLE II at conference in India
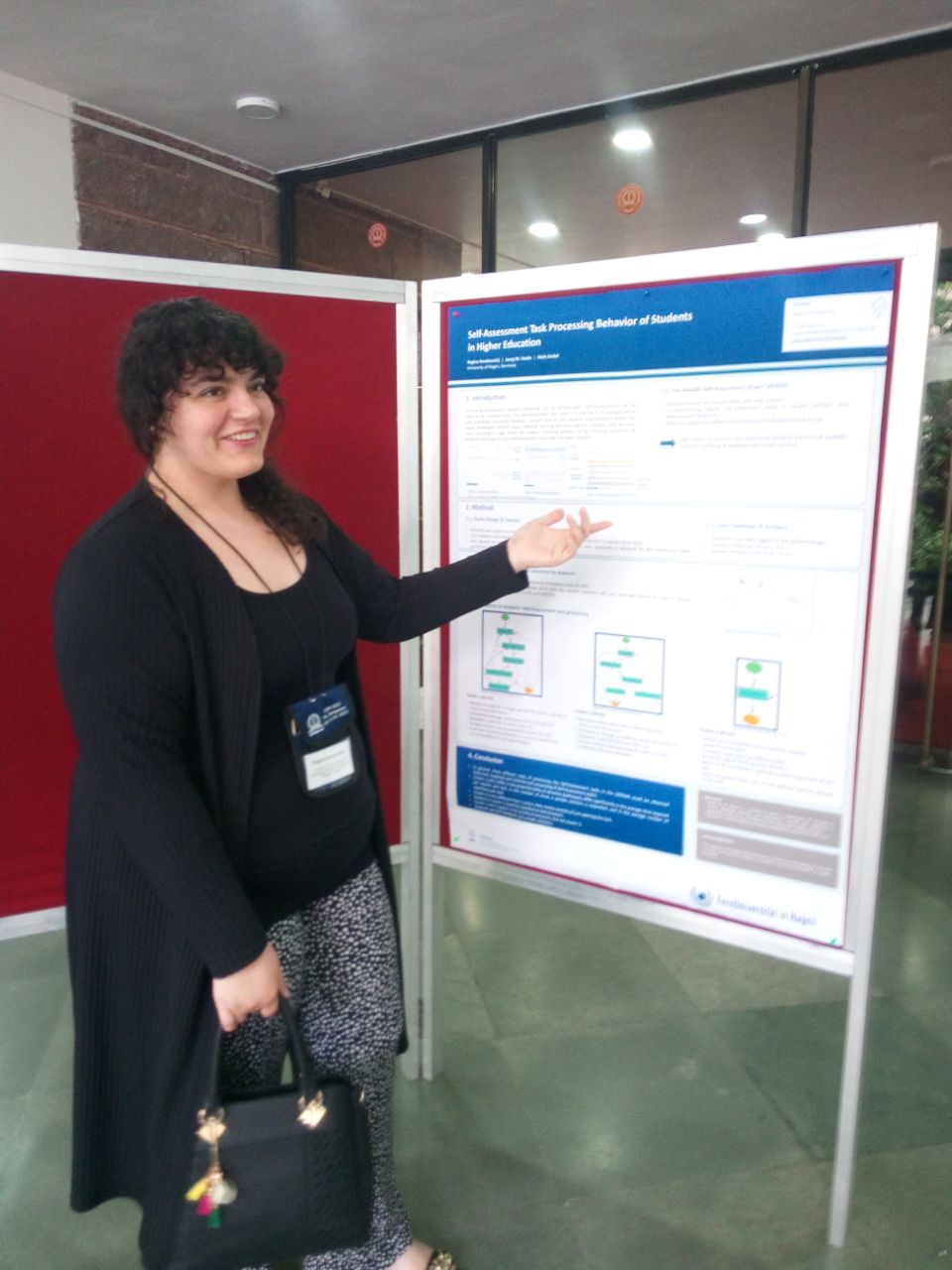
CATALPA junior researcher Regina Kasakowskij recently presented results from the APLE II project in Bengaluru/India at the Educational Data Mining 2023 conference. She is working on a self-assessment tool for her dissertation in the APLE II project. The aim is to give students adaptive feedback on their task solutions. The feedback takes learners' individual preferences and past learning behavior into account. To this end, she developed SAFRAN - a plug-in for Moodle.
SAFRAN stands for Self Assessments with Feedback RecommendAtioNs. Students can use it to self-assess their solutions to exercises with the help of assessment criteria and then receive appropriate feedback. "What's new about the tool is the combination of situational feedback, a feedback option for students on this feedback and the opportunity for teachers to set scalable complex tasks in a digital learning environment. Thanks to its ease of use, the plugin particularly encourages students to repeat and practice tasks several times," says Regina Kasakowskij.
In order to determine how well the tool supports students in self-assessment, the researcher also investigated how sequences of learning steps during task use affected the scores achieved. "We found that both students who solved the tasks very quickly and students who spent longer on individual texts or tasks received similarly high scores to those for tasks that were assessed by correctors. First of all, one might think that the first group may have only guessed the criteria for evaluating their solution because they were so quick to complete the task. However, a comparison of the scores shows that they were actually just good. For personalized feedback, this in turn means that we should also focus feedback on suggesting more advanced or more difficult tasks to these fast students using computer-based adaptive testing, for example," explains the researcher.
Regina Kasakowskij's tool was well received at the conference in India. She is already in contact with international colleagues who would like to use the tool for their own research and teaching.
Niels Seidel and his team are currently working on this as part of APLE II:
- Introduce learner models and adaptation rules for the definition and automatic generation of feedback (e.g. with LLM) on learning processes within a lesson (Niels Seidel);
- concept maps and other types of tasks for the adaptive assessment of reading comprehension in parallel with the reading of study texts (Dennis Menze);
- to evaluate the support of self-regulation in different online learning environments with the help of a theory-based classification system (Slavisa Radovic);
- to investigate the connection between self-regulation support and learning behavior in a longitudinal section (Slavisa Radovic);
- AI-based automated dubbing of multifaceted study texts and making them usable collaboratively as hyperaudio (Niels Seidel).
Current events with Niels Seidel